MIMicking Human Audio, Speech and Language behavior with AI
We are a team of machine learning and speech & language scientists studying human communication, perception and behavior. More specifically we focus on speech in noise perception, but recently we have also drawn our attention to other aspects of human communicative behavior that may be modeled using modern machine learning techniques, such as written language. We have also started focusing on other applications of mimicking human behavior with machines, spanning from navigating environments with teleoperated robots, to listening bioacoustic audios in search for particular species calls.
Our project has two goals:
- make machines recognize speech and other tasks more like humans do
- validate our understanding about human speech perception through the use of data-driven techniques
MIM aims at proposing computational models that predict human speech recognition at a fine resolution. Current approaches to intelligibility prediction provide macroscopic estimates consisting of aggregates over many stimuli and listeners. By leveraging recent developments in the field of Artificial Intelligence, models could predict recognition at a sub-lexical level. Deep learning (DL) has improved automatic speech recognition performance significantly, achieving super-human transcription in conversational tasks. We plan to build DL models to predict human listening tests responses aiming at improving individualization of hearing solutions. Scarcity and variability of human listening data, and the interpretation problem in DL are two of the main issues that we will tackle.
The project is funded by an ANR JCJC grant ANR-20-CE23-0012-01 for 4 years from September 2021.
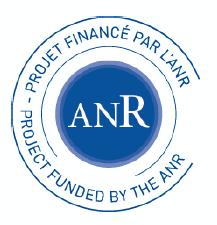